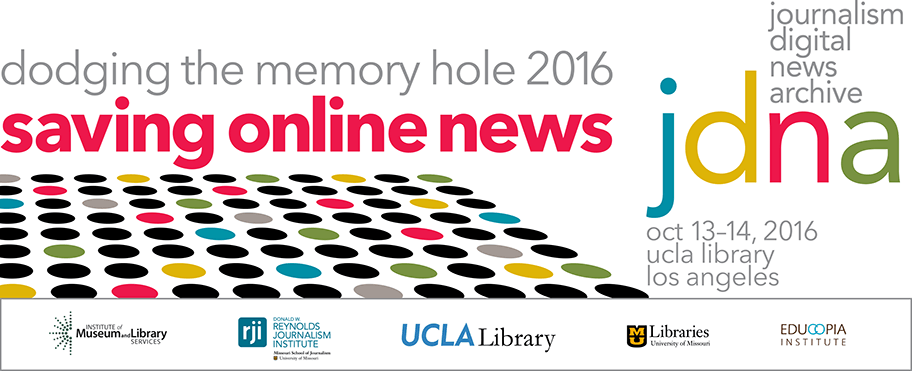
Michael Nelson: Presentation: Summarizing archival collections using storytelling techniques
Scroll to view transcript
MICHAEL NELSON: [00:07] So, what I’m going to be talking about today is Yasmin [AlNoamany’s] Ph.D. work.
[00:11] So, if you ask me any difficult questions I’m going to deflect the answer to her; she’s not far away at Berkeley doing a postdoc there. So, what I want to talk about is utilizing storytelling techniques to enrich archival collections. All right. So, this is really work with Archive-It. And Archive-It, as most of you are aware, is a subscription service that allows its members to build themed collections. So here’s the home page, and we have the stats there, probably always out of date.
[00:47] Lots of collections, thousands of collections, many institutions and literally billions of archived pages are what we call mementos. We go to a particular collection, this is the 2013 Boston Marathon bombing, and we see some metadata. Some of this coming from the creator of the collection as well. Up at the top, we have a description. Here in the middle, we have some of the seed URIs that the creator of the collection decided was important and put them in the collection. And then also from the creator of the collection, we have some topics and other descriptive metadata that describes what we can expect to find in the collection.
[01:29] But one of the issues with this is the number of collections proliferate. We have this weird scenario where the larger the collection is, the harder it is to explore and find out what’s in the collection. And as different collections pop up it’s hard to differentiate one collection from another one. So, Yasmin is from Egypt, the Egyptian revolution happened while she was in Norfolk, Virginia. So all of the — almost all of the examples are going to be themed around that, and her creating collections and exploring the web archives for her son, who was too young to really understand what was happening at the time.
[02:10] There are at least three collections on Archive-It that have something to do with Arab Spring or the Egyptian revolution. And here are screenshots on them. So, how do you explore what’s in which collection? How can you differentiate between these collections? Even worse, in a manner of speaking, this is one of seven human rights collections. I think there might actually be more now and it becomes really difficult to understand what’s in which collection. So, this one is coming out of Columbia (University). So, we still have the descriptive metadata, we still have the topical information, but we scroll down and we even have descriptive metadata about the seed URIs.
[02:50] And then we decide one of the seed URIs is interesting, and then we get essentially just a listing of how many times it’s been crawled. Now, if you’re already familiar with that collection this maybe is all you need; but, if you didn’t create this collection, then you don’t know. Right. This is like the stuff that’s in your attic or your basement. You put it there, but over time you sort of lose track of what exactly is there. Sort of the ski boots that get thrown away, right?
[03:19] So, we tried earlier attempts, some standard visualization techniques to get a better understanding, to differentiate one collection from another. So we have tree maps and timelines and lots of pretty pictures that look great on the graphs. They make for great demos, but they really don’t help you understand the difference between one collection. And the problem was it was focused on trying to visualize everything. This works with small collections, but once you have thousands of seed URIs and potentially thousands of mementos of each one of those seed URIs, you have to let go of this idea that you’re going to try to visualize everything. What we really need to do is sample and come up with just a small sprinkling of what’s important from the collection and use that as a summarization.
[04:09] So, one of the ideas that we became fascinated with was the idea of storytelling. Now, that sounds cool and it means a lot of different things. So, we’re here in the library. We probably have some humanities people. Storytelling for you means this and that’s great; we have characters and they go on a journey and can encounter conflict and there’s resolution and we learn something in the end. That’s great. That’s not what we’re talking about. Storytelling in social media is basically arranging pages in time. Right.
[04:42] And so we see this with, Facebook has the Look Back, the Year in Review, and there are Twitter stories. There are other applications like — anyone use 1 Second Everyday? Where, it has this idea that you sample small bits of your large personal collection to try and create a summary of what’s in there. So that’s what we’re based on. We’re going to mostly talk about Storify, because it has a really nice interface, but realize that the storytelling idea is represented across a lot of social media tools. So, we’re going to look at Storify, but if Storify goes out of business, or God forbid, Apple buys Storify and kills it like they did with Topsy, then we could probably shift to another interface.
[05:29] So, great! We have storytelling. We have these archival collections. What do we do about it, right? Why don’t we just use Storify? Well, it has some limitations. Yasmin went and found this story in Storify about the Egyptian revolution and, of course, you know what’s coming, right. Click on the first thing and it’s 404. So, Storify and all of these things are really nice but they’re kind of just fancy ways to arrange bookmarks and there’s no actual preservation behind it because, of course, nobody thinks about preservation.
[06:00] So, what we really want to do is take the collections that we have in places like Archive-It and then combine them with a storytelling interface. And the idea is, I’m not going to try and tell you that Storify is the best available interface, but it’s the interface that people already know how to use. And, one of the lessons that I’ve learned in computer science is, at some point you have to give up trying to convince people about the right way to do it and just let them use whatever tool, and you adapt to the tool that they use. So, for example, email is the stupidest way to move files around, right? But we do it all the time. I’ll send you the slides over email.
[06:41] That’s a terrible, terrible thing, but OK I’ll send them to you over email, right. I’ll stop trying to convince you about… Now, this gets better with Dropbox and other things, right, but you know what I’m talking about. So, what we’re trying to do is come up with these archive-enriched stories, and have an exploratory interface in a social media storytelling environment that users are already familiar with. So, basically it works if you consider the collections that are in Archive-It, if you consider those as samples of a much larger web. So, somebody picks out the seed URIs, conducts the crawl and decides that this is what I want to build a collection on.
[07:23] Really, the stories that we’re going to generate are essentially doing the same thing, but are sampling from the collections. So, there’s a continuum of going from large to small, as we go from left to right. These were some stories that Yasmin handcrafted about the Egyptian revolution, sampling from the collections in Archive-It. And we’ll come back to these. So, basically we have different kinds of stories that we explored and the URLs; I’ll post the slides later so you can gain access to all of this. But these were the hand-crafted stories that she created. And, what I’m appealing to is, intuitively, this is a better interface for exploring the collection than the Archive-It interface.
[08:05] Now, this is not everything that’s in the collection, but this will allow you to differentiate one collection from another. So, generating this by hand is difficult. Yasmin was motivated to do it for the Archive-It collection, but what about the 3000 other collections that are there? How do we do this automatically? So, first we want to discuss that collections really have two dimensions. So we have time, and this is real-world clock time, right. Then we have different URIs, so these would be the seed URIs, and we have some kind of sampling through time. Now, sometimes the page stays basically the same, sometimes the pages change.
[08:43] So, we have a lot of variety in here, but we basically have two dimensions. If we take the cross product between these two dimensions at a high, rough level, we have basically four kinds of stories that we can tell. The first one is it exists, but it’s not really a focus. Basically you could have the same page at the same time, but vary based on things like mobile versus desktop, or GOYP or something like that. So this exists, but this is not the kind of story we’re going to create in the collection. Another kind of story we can do is have a fix page in sliding time, so you could pick BBC or CNN and then just have the samples track how the story is being reported.
[09:27] And this gives you an idea — especially for something like the Egyptian revolution — of what’s happening through time. Then we can have a sliding page, fixed time. So the idea is we pick some time that’s interesting. January of 2011, Mubarak steps down. And then we can talk about how that news story is reported at different venues. So, we have the BBC page, we have the CNN page, we have all these things and we can get different perspectives of how things are being reported.
[10:03] And then there’s sort of probably what you thought of as the most standard way, the sliding page and sliding time. Basically, we’re sampling from different seed URIs at different times and basically using this to summarize. So, we have a progression of the story, and we also have multiple points of view here. So, we’re storytelling and we need a name for a framework. Obviously, we call this the dark-and-stormy archive framework. [Laughter]. I’m glad you laughed. I had to explain it to Yasmin. She didn’t grow up with Snoopy. [Laughter].
[10:35] So, basically the first thing we’re going to do at the top is really try to understand what’s in social media collections, try to understand what is an Archive-It collection. And then we’re going to review a set of methods to go from thousands, if not millions, of pages trying to get down to just a handful of pages that we can push into Storify. All right. So, the first thing, figuring out a baseline of what people put in Storify. What does social media look like? So, there are a bunch of questions we can ask. What’s the length of the story? So here we have a story that someone created about the Boston Marathon bombing. If you scroll down you find this has 31 things in it, 31 resources.
[11:21] Then we can try and figure out what kinds of resources. So, you can add text. You can post videos and have tweets and webpages and so forth, trying to get a feel for what kinds of multimedia resources people want to include. Then, we can look at domain distribution. And it probably won’t be a surprise to find out that Twitter is sort of the dominant domain that people are using in Storify, because people go on these tweet storms and have 37 tweets about a topic and then somebody will arrange them into a story, so, they’re all clustered and grouped together.
[11:58] We downloaded, using the Storify API, we downloaded a whole bunch of their stories, ran some analysis, and this is the top 25 domains. Basically, Twitter is dominant with 82 percent of the domains. Then it falls off pretty rapidly, but it’s still basically all social media — your Instagram, YouTube, Facebook, Flickr; some other stuff — Blogspot, WordPress, a variety of blogging and social media platforms. And then it’s a long tale of basically everything else. So, then we wanted to understand what constitutes a popular story, and we’d define popular stories as having in the top 25 percent of the views normalized by the time available that it was on the web. This is a story about the shooting in southwest Virginia, Roanoke. It was 19, almost 20,000 views. And this story has 64 views. How do we tell these apart? We analyzed a bunch of characteristics of the story. Basically, the punch line is we could tell the difference.
[13:10] The popular stories had a median of more resources. They typically would have 28 resources, instead of 21 resources, and the editing time that people spent putting those stories together was significantly longer. And then we also looked, and this is related, that the link rot of the elements that were in the story was significantly less,10 versus 15 percent, for popular versus unpopular stories. So, basically the popular stories reflect somebody performing a curatorial activity; adding more stuff, continuing to edit it, perhaps replacing broken links with non-broken links or perhaps choosing better links to begin with.
[13:53] So, one of the takeaways here that we operationalize in the future is [that] this gives us a target. If we’re going to automatically create stories, they should start to look like human stories. And 28 was the median number for popular stories. So, this gives us a target. If we’re going to create a story automatically, let’s shoot for 28. Too few elements in the story and you don’t get a good summarization; and, too many resources in the story, then you get a too long, didn’t read. So, there’s a sweet spot for a number of things in there. Then we also did a baseline of the Archive-It collections.
[14:31] And, again, here’s a collection; this is the Egyptian revolution. And, for example, this has 435 seed URIs. And then we looked at the mean and median of the mementos of the seed URIs and, for example, this one’s got 16 resources. Then we looked at most frequently used domains. In this collection, the Boston Marathon, we have ABC News and blogspot, and these were top seed URIs in this collection. Skipping a bunch of data, the takeaway message here is the Archive-It top 25 hosts really look nothing like the Storify top 25 hosts.
[15:15] So, the number one slot here is the publications of the government of Canada. Now, they’re Archive-It members, so obviously they’re archiving their own material. So that’s 40 percent of the content that we found in Archive-It. Now, it really only spans four collections, so obviously government publications are not super popular outside of Canada as you might imagine. We skip down, and Twitter is number 10. So, we’re not really archiving a bunch of tweets or things.
[15:50] Twitter does not show up in the Archive-It collection. So, it’s basically one percent of the total collection, although it does spread out over 460 collections. So, there are a lot of collections that have the random tweet thrown in there, but it’s not this dominant force that we find in Storify. So the takeaway message here is what we archive in Archive-It — now, remember in Archive-It, we essentially have professional librarians, people who are archivists, people who have an idea about, We should be collecting these resources for posterity.
[16:37] What we archive and what we share on social media are completely different. So, seeds and shares are not the same thing, and there’s been a bunch of publications that kind of find this same result over and over again. But what we’re archiving and what we’re viewing on social media are different. So, to create these collections, one of the things we’re focused on was detecting off-topic pages, because this is something that can happen. Archive-It gives you really nice tools for performing a crawl and understanding what happens in the crawl, but basically the data that you get back is about HTTP events. You had this many hosts and this many 200s or 404s and this many MIME types and so forth.
[17:07] It doesn’t give you an idea about the content. So, Yasmin went through one of the collections, and this was a candidate for one of the the first Egyptian election. This was his home page and this was a seed URI in the collection. Then, shortly after this, they had a database error, and these were all 200s, so it is not detectable as 404s. And then they ran out of money and then — apparently in Arabic, this says we ran out of money, I trust that she’s telling me the truth on this — then it came back on line, so you can’t just say, “OK, you’ve gone off topic, you’re off topic forever.”
[17:42] That oscillates back and forth in time. Then they’re are hacked, and the English says they’re hacked and there’s some Arabic. And then they lost their domain and it’s for sale. So, all this shows up in the collection. Now, we’re not talking about removing this from the collection, but if we’re going to create a story that summarizes what’s in the content, at some point these are not good candidates to show up in this story. We really want to detect and focus on these as representative of what the collection holds. We can automatically — she did this by hand — we can automatically detect off-topic pages.
[18:17] So, one way is to use a suite of textual methods. Here’s one version of the page, and then it’s changed, and then you can use cosign similarity. You can use Jaccard or T.F., a term frequency intersection. It doesn’t really matter about the details, but, basically, intuitively, these look the same and the scores sort of reflect it. And you can sort of empirically determine where the thresholds are. When you compare this to that, obviously the number of terms in this case falls to zero. So, we can use textual-based methods to figure out, what are the subsequent changes, are they still somehow similar to the original page. But, sometimes the page is still on topic, but straight lexical comparison of terms won’t find that for you.
[19:05] So, some of the nouns from this page, Mubarak to hear square; violence army; and then Egypt protests Morsi Cairo President. This is clearly about the same stuff even though the words don’t overlap. Then there’s a technique where you submit these as a query to Google. You get the snippets, and this is like a cheap and easy way to do term expansion. Then you’re really counting on these terms appearing in the snippets here, and then you apply the textual results; and if they overlap, then you decide it’s still more or less on topic. It turns out there’s actually simpler ways to do this.
[19:40] We can look at the change in the size, either in the number of terms or in the number of bytes that come back. So, here’s one version of the page. Here’s another version of the page.
Visually, looks very different, but it’s roughly the same size page. Legitimate pages have a target page size. In this case, when it says there’s a database error, we notice it’s significantly smaller. The page has greatly shrunk in terms of the number of terms or the bytes that are sent back for just this page, and that’s a clue that something’s gone wrong.
[20:14] So, we have we — this really means Yasmin, she worked really hard on this — created a gold standard data set for three collections: Occupy, Egypt revolution, and Columbia Human Rights collection; and went through and manually labeled off-topic momentos. So, seed URIs, total momentos: 458 off-topic; here, 384 off-topic; and, here 94 off-topic. So, basically, we went through – she went through – and manually labeled these. Then we combined individual methods and combinations of methods and basically came up with the best approach figuring out on the gold standard data set of how we could automatically tune and detect off-topic pages.
[21:01] Then we ran it on 18 collections and tried to discover off-topic momentos in those collections. We got pretty good results. We can’t tell the ones that we missed, but we can examine the ones that were flagged as off-topic. Then, we went and manually determined if they were truly off topic. Now, some of them, some of the collections have no off-topic pages that we could detect. So, that’s great. Some of them had small numbers at the top and then, you know, here’s a Southern California wildfire web archive, and we did really badly on this one.
[21:35] So, we flagged 300 and some, but only 200 and some were actually off-topic. Mostly things are on-topic in the collection, but it’s not uniform across. So, if we remember we’re trying to pick 28. Now if we exclude the off-topic pages, that gets us a lot of the way there. We thought we would find distributions like this where we have time in seed URIs and we have a highly regular crawl schedule. Turns out that’s not the case. We have wildly irregular crawl schedules.
[22:12] This gets tricky to try and pick 28 in this dimension and that dimension, and we’re going to skip a lot of the details of the clustering. But basically we figured out a way to try and get to 28. To try and pick candidate pages, we want to pay attention to the quality of the page. There’s lots of dimensions of quality that we consider. One is looking at the amount of resources that were missed when it’s crawled, but it’s weighted toward sometimes you miss important resources and sometimes you don’t. Justin Brunello, we leveraged his Ph.D. work where it’s not just straight percentage of “I got nine out of 10 so it’s 90 percent.”
[22:57] If that one was the main video in the middle of the page, then that was important and your damage is greater than 10 percent. So, basically we want to prefer this page over that page because this one is visually less damaged and we can figure this out dynamically. And, they kind of say the same thing, but given a choice, prefer this one. Since we’re headed towards Storify we actually prefer pages that generate more attractive snippets.
[23:23] So, if you look at the top, you’ll see that this snippet and Storify automatically gets the image; 25 prettier than this; and that actually is going to matter in this interface. Even though they kind of say the same thing, we want to choose the page on the right. Basically, this can be operationalized by – if you’re choosing deep links into the collection, you’re actually more likely to get more attractive, more meaningful snippets because the metadata is specifically about the page, and the snippets that come from high level pages, even though the story might dominate about whatever the metadata is just, “BBC is a great news source or something like that,” and doesn’t help distinguish one page for another.
[24:06] As a footnote, it turns out social media snippets, social media pages don’t often generate good snippets. We don’t have that many in Archive-It collection anyway, so it doesn’t matter. So, we go through, we pick 28, we push them into Storify, and here are the handcrafted stories that we saw earlier. If we zoom in, it’s kind of hard to tell, but the Favicons, they all say they come from Archive-It, even though they’re originally crawled from different locations they don’t always have nice images and the titles aren’t so nice.
[24:41] So, basically you don’t get this from the Storify user interface, but they have an API where you can overwrite the Favicon, you can add the date to the title and you can modify the metadata and essentially get more attractive stories. Here, the Favicons, it’s saying this comes from National Post, BBC, New York Times, etc.; and essentially we all have the images. We have the dates and the title. We’ve created a more attractive interface that you don’t get from just copying and pasting.
How do we evaluate? How do we know if our stories are good? So, evaluation is tricky. There’s no Voight-Kampff test. I’m in Los Angeles.
[25:20] I have to mention “Blade Runner.” So, basically the idea is we’re going to use Mechanical Turk and we’re going to compare the automatically generated stories to those hand-crafted by experts at Archive-It, and if the Mechanical Turk workers can’t tell the difference between the automatically generated version and the human generated version, then we’ll consider them just as good because otherwise, if you’re picking 28 out of millions of pages, there’s no way we can pick the same 28 but we can have equally good summarizations. So, we gave some criteria to the people at Archive-It. You can read that later.
[26:05] They created stories, 23 stories for 10 different collections. Now, a lot of these, or basically all of these, are news-oriented collections. This is an important distinction. I mean, it’s obviously important for this venue, but it’s not clear that this technique will work for the state government websites and some of the other aspects that show up in Archive-It. But, you guys don’t care about that, so we’re good. Then we have different kinds of stories. This is a sliding page; sliding time, fixed page and sliding time. Now, for some collections, we only got certain kinds of stories, so it’s not a full 30 stories but in some essence, intuitively, this is the most interesting kind of story that we want to examine.
[26:45] So, here is a page generated for the Boston Marathon bombing by the experts. This looks good, and we have the good Favicons, the good titles and so forth. Then, we had a process, I’ll skip over the details, but there’s lots of clustering and throwing out duplicates and off-topic and so forth. This is the automatically-generated page. This is clearly different, but intuitively, it kind of looks the same. You can’t really tell who generated which. We generated random stories and basically we just picked 28 things randomly from the collection and it’s not bad.
[27:21] You get some stuff, but you get, “Welcome to city of Boston” and so forth, so you actually get some bad stuff that’s thrown in there as well. Then, as a control, we generated some bad stories, which basically the same memento picked 28 times over and over again. If a Turker said, “I like this story,” we said, “OK, then we don’t like your data.” Then, we ran a comparison where we had three different kinds of hits where we compared human versus automatic, human versus the poor pages and so forth.
[27:56] We only used master-qualified stories and we threw out, if you picked the poor stories, or if you required less than seven seconds, which is clearly not enough time. The median time was actually seven minutes – they spent a lot of time – and 50 cents for a hit is actually a pretty generous hit amount. So, this is what a hit would look like. They would have a left and a right. It didn’t talk about human versus automatic. We said, “We generated stories. Which one do you like best?” And these were scrollable, independently scrollable. They would pick it. The punchline is: preference for automatic and human stories is basically indistinguishable. They couldn’t tell the difference between a human and automatic story, but they could tell the difference between a human and a random story, and an automatically generated and a random story.
[28:44] So, random is not equally good, but the experts versus automatic are equally good. Now, for different kinds of stories, sliding page sliding time, etc.; basically, they came out the same. This also holds versus the random and automatic versus random. So, we basically didn’t see a distinction between different kinds of stories. They were all easily identifiable. So, the punchline here is the automatically generated stories are just as good as the human stories.
[29:18] Now, what we didn’t prove is that these stories are useful for anything at all. It could be that they’re equally bad, and I get that; but, at least they’re indistinguishable, so that’s a start. And, the summarization here is, return to the original slide, is we’re taking the Storify interface people already know how to use, sampling from the Archive-It collections, and using this to create a summary of what’s in the collection. I reference a bunch of data sets and code and papers and slides; and basically everything is available right here. And I think that’s it. Thank you.
Comments