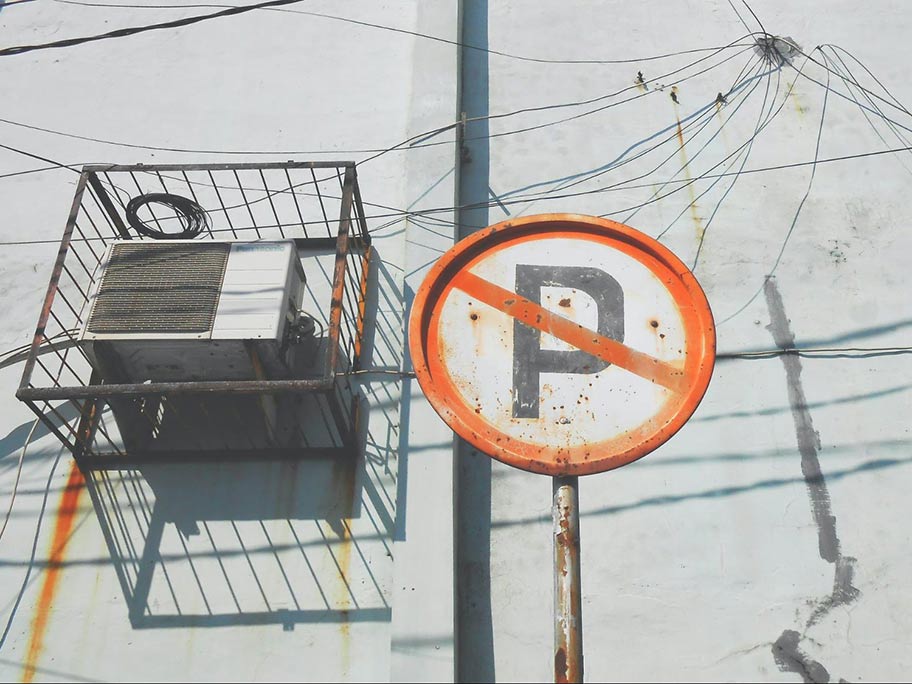
Photo: Jimmy Ofisia, Unsplash | https://unsplash.com/@ofisia
When journalists should care about p-values
And when they shouldn’t
There’s a saying in statistics: “All models are wrong, but some are useful.” In other words, it’s a little quixotic to imagine we can capture the nuances of reality with simple models, but some of these models can make the complex more legible. The first chunk of the saying is the important bit, though. Most models are wrong. It’s not just true of statistics: A 2005 study of scientific reproducibility—that is, the ability to replicate findings reported by researchers—went as far as to argue that “it is more likely for a research claim to be false than true.”
Most published research is “false” in another, more subtle sense: A lot of science is based on what’s known as null-hypothesis falsification. Researchers—in particular, biomedical researchers, psychologists, and social scientists—start with an assumption about the world. The assumption is a boring one; a statement along the lines of “There’s no medical difference between this experimental drug and this placebo” or “There’s no relationship between cities’ rental costs and their rates of per-capita homelessness.” This claim of ‘no effect’—the null hypothesis—is that which must be statistically rejected if the researchers are to say anything interesting about the world. (Things like: “The drug works” or “Rent predicts rates of homelessness.”) Research is about falsification.
As data and science journalists, it’s often our job to review and report on published research. Certainly, journalists should know how to read falsification-based science. They should know how to interrogate papers grounded in null-hypothesis significance testing—know how to sniff out bad science in the same way they should know how to sniff out gas leaks in their homes. But, I argue, they should not limit their own quantitative research to the same statistical realm, and they shouldn’t halt their investigations because some puny statistical test didn’t hit some predetermined significance level. (“Absence of evidence is not evidence of absence.”) They should know how to construct useful models.
And so we arrive at the p-value.
You’ve seen these things before, usually shrouded in parentheses and expressed as inequalities (p < 0.01). They’re those tidbits that tell you whether you can allegedly trust what you’re reading.
An assertion: The p-value is the most twisted, readily misinterpreted, easily misapplied statistical nugget on the face of Gauss’s green statistical earth.
Here’s why. A p-value is a probability. (That’s what the ‘p’ is for.) In particular, after a researcher summarizes a dataset by computing some statistic (say, the mean of a group of values), the p-value corresponds to the probability of observing a summary statistic at least as extreme as the one they’ve just calculated, given that the null hypothesis—the claim of ‘no effect’—is true. It’s the statistical version of innocent until proven guilty. If you’re going to reject the null hypothesis (i.e. the presumption of innocence), you need to demonstrate that the circumstantial evidence before you is extremely unlikely to have arisen under that assumption.
In other words, here’s what a p-value is not:
- It is not the probability that the null hypothesis—the claim of ‘no effect’—is true.
- It is not the probability that an alternative hypothesis isn’t true.
- It is not the probability that the result you’re observing is due to chance.
- It is not the probability of mistakenly rejecting the null hypothesis.
- It is not the probability that you’re wrong.
Those last three are particularly insidious, because they map onto a lot of our colloquial understandings of p-values. Importantly, p-values operate within the world of the null hypothesis. They can only say things about that world. Look back at the technical definition above: “given that the null hypothesis is true.” Calculating a p-value means assuming a lack of effect—in whatever specific manner that assumption is crafted—summarizing the data in front of you with a test statistic, and asking how likely it is that a test statistic that extreme might arise, given your assumption of the null hypothesis. That’s a very specific statement, and it says nothing about the likelihood of alternative hypotheses, the likelihood of the dataset before you, or the likelihood of the null hypothesis itself.
In psychology and the social sciences, we tend to understand statistical significance as a p-value of less than 0.05 (i.e. a 5-percent chance of observing a test statistic like yours under the null hypothesis). In a lot of the biomedical sciences, that threshold drops to 0.01 or 0.001. A colleague in the public sector once told me their legislative cutoff for statistical significance was 0.2. These thresholds are awfully arbitrary. But they’re made even more so by the fact that we’ve decided to drape the vaunted moniker of “statistical significance” over an idea that captures such a specific aspect of our data.
Yet studies are awash with p-values, and so we’d best understand what it is they do. We can also ask ourselves some critical questions when we come across them. Importantly, when reading a paper that deploys p-values to prove a point, consider asking yourself:
- How convinced am I? What null hypothesis is being rejected here? Is it a reasonable null hypothesis to reject, or is it a strawman?
- Might some other model explain the data? In other words: Sure, the researchers have rejected a null hypothesis, but are other alternative hypotheses consistent with the data they’ve presented? Rejecting a null hypothesis isn’t the same thing as confirming your preferred model.
- Have the researchers corrected for multiple comparisons? A p-value of 0.05 suggests that 5 percent of the time, a test statistic as extreme as the one under examination could have arisen under the null hypothesis. If they run 20 tests, by their own definition of statistical significance, one of those tests should be a false positive! (Ask enough statistical questions of a dead salmon performing a psychological test, and it’ll look like its brain is lighting up.)
- Does the study in question have sufficient statistical power? A power calculation examines a bit of a flipside to the p-value coin. It asks, given that the null hypothesis is false—the opposite of what’s assumed in a p-value calculation—what’s the chance you’re correctly rejecting it? What’s the chance that the statistical test you’re using isn’t giving you a false negative?
These questions are useful for guiding our reading of quantitative studies, because most researchers deploy p-values as their central statistical reference frame. But my larger point is: It doesn’t need to be your statistical reference frame.
Instead, you can choose to build an understanding of model plausibility from the ground up. Plausibility is all about counting: about counting the number of ways the data we observe in the world might arise under a variety of competing possible models. To say that something is ‘more likely to occur’ merely implies there are more chances for it to happen. Whole subfields of statistics rest on these conceptions of likelihood and plausibility, and within them, p-values are nowhere to be found. I find these methods infinitely more convincing. They’re more flexible, require fewer assumptions, encourage greater creativity in analysis, and respect the complexity of the real world.
Sure, in part, maybe this post is a cry into the void for more data journalists to be Bayesian statisticians. (Heard that phrase tossed around and want to know more? Richard McElreath’s textbook Statistical Rethinking is a masterclass in… well, exactly what the title says.) But more earnestly, it’s a wish for more art and intuition in our analysis. Null-hypothesis significance testing is profoundly uncreative—and when you don’t follow the rules, you risk reaching unfounded conclusions. Science (and data journalism) should be about convincing yourself that the effect you’re observing couldn’t have arisen by any other means than the model you’re reporting on.
Forget the arbitrary rigidity of the p-value and the null-world it describes. Falsification is supposed to be about proving yourself wrong: Science advances when you can no longer do so.
Significant? Insignificant? You can get in touch with Clayton Aldern at caldern@grist.org on Twitter @compatibilism.
Comments