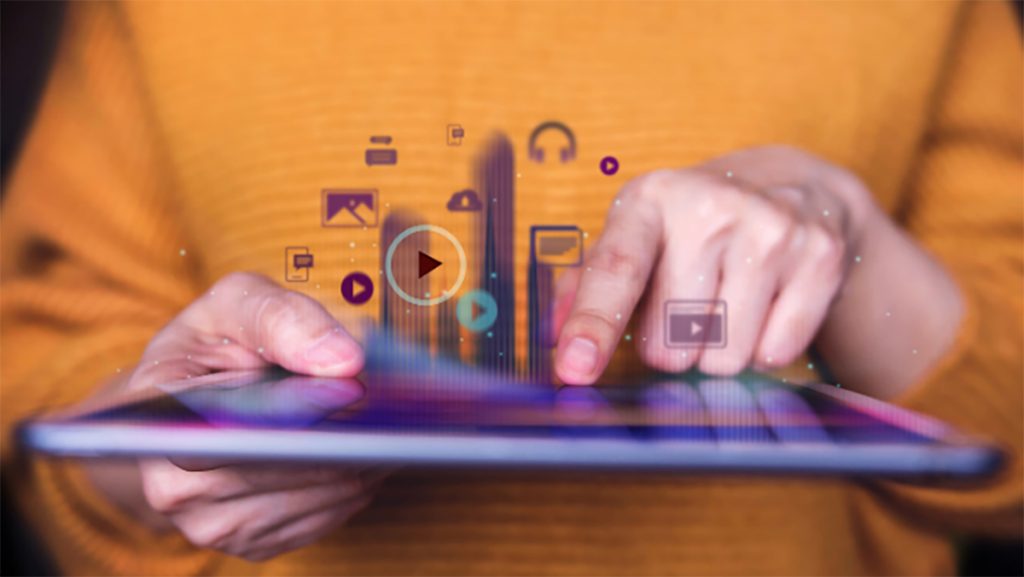
Photo: BlackSalmon | Canva
Big tech algorithms: The new gatekeepers
Algorithmic Literacy for Journalists will be an interactive resource to help journalists understand the functions, consequences and ethics of algorithms in a digital age
With algorithms classifying, prioritizing, and filtering information online, driving content recommendations made by search engines and social media feeds — algorithms are not only reshaping how news is distributed and consumed but also how it is gathered and produced.
Developed, implemented, and held in secret by Big Tech companies — including Google, Meta, and X —proprietary algorithms function as the new gatekeepers, determining which news stories circulate widely and which are buried from the public’s view.
This reality raises serious questions about transparency and accountability in determinations of newsworthiness, and poses a host of practical problems for journalists, editors, and news outlets.
One consequence is that online “likes” and “shares” have become new currencies that journalists and editors are tempted, if not forced, to chase, leading to concern that promotional motives and pressures will preempt journalistic values and craft.
But a deeper concern involves how the new, algorithmic gatekeepers filter news about politics and social justice issues. For example, digital news aggregators, such as Apple News and Google News, have been found to skew towards soft news stories drawn from a narrow range of sources, and to highlight biased content, including stories that reflect and promote anti-LGBTQ prejudice.
In 2020, when Emil Marmol and Lee Mager surveyed the “deplatforming and de-ranking of dissident, counter hegemonic, and alternative media voices, from the libertarian Right to the anarchist left,” they found dozens of cases in which alternative, independent news outlets covering racism, environmental degradation, class struggle, illegal surveillance, violence by state actors domestically and abroad, and corporate malfeasance were subject to online censorship.
Amidst the relentless onslaught of information and range of perspectives online, it is convenient (but incorrect) to assume that search engines and social media feeds are neutral conduits of information and perspective. That assumption blinds us to the pitfalls of algorithmic bias and suggests why algorithmic literacy is a vital component of 21st-century media literacy.
Many Americans are unsure whether Google News, Apple News, or Facebook do their own reporting, further illustrating how the impacts of algorithmic recommendation systems on news are subtle, if not completely invisible, but also consequential to the public’s access to news and information.
With these concerns in mind, I agree with Mandi Cai and Sachita Nishal of Northwestern University, who have asserted, “Every journalist, no matter their specialty, should be AI literate.” Their call motivated the project I am pursuing as a 2024–2025 Reynolds Journalism Institute Fellow.
What I’m building
Algorithmic Literacy for Journalists will be an interactive resource to help journalists navigate this reality. This resource will help develop journalists’ ability to understand the functions, consequences, and ethics of algorithms in a digital age. And a core focus of the project will be helping independent journalists and newsrooms whose digital content has been subject to shadowbanning, demonetization, and other forms of online speech filtering that restrict them from reaching a wider audience.
Most working reporters and editorial staff lack the time or the training to fully appreciate the impacts of algorithms and AI on their daily work routines. Nevertheless, through search engines, social media platforms, and online databases, algorithms shape — and sometimes filter or block — the flow of newsworthy information and opinion. One basic aim of this project is to help journalists become more savvy about specific ways that algorithms influence the creation and distribution of their work.
This resource will help journalists and newsrooms whose digital content has been subject to shadowbanning, demonetization, and other forms of online speech filtering that restrict their content from reaching a wider audience. It will walk journalists through how to identify and address these types of online speech filtering with step by step templates, guidance and examples.
Future articles on the progress of this project will focus on how algorithms affect story production and distribution and what journalists can do about this.
Working toward algorithmic accountability reporting
Better coverage of artificial intelligence would enhance public understanding of AI’s impacts on the everyday routines of ordinary people, but it could also help inform the public about how AI is transforming journalism. Although social scientists study the topic, reporters and their editors seem to shy away from reporting on media policy and other political factors that impact the production and distribution of news.
In 2022, Sayaash Kapoor and Arvind Narayanan, computer scientists at Princeton University, published a list of eighteen pitfalls in AI journalism, organized into three related categories: flawed comparisons of AI systems to humans, hyperbolic or incorrect claims about AI, and platforming sources with self-interest. Regarding the latter, Cai and Nishal, the Northwestern researchers, noted that AI news coverage is “saturated with industry perspectives.”
What’s needed is “a reorientation of the traditional watchdog function of journalism toward the power wielded through algorithms,” which Nicholas Diakopoulos, a data science researcher at Northwestern University, called “algorithmic accountability reporting” in his 2019 book Automating the News. Diakopoulos focused on “a new strand of computational journalism,” but his concept arguably has wider applicability to a variety of story types and investigative methods united by their focus on “how and when people exercise power within and through an algorithmic system, and on whose behalf.”
One pioneering example of this type of reporting, cited by Diakopoulos, is ProPublica’s Machine Bias series, initiated in May 2016, investigating racial bias in the algorithms used to calculate risk scores in criminal sentencing.
Adaptations and solutions
Here are a few initial recommendations for better journalism on AI based on published research:
- Avoid reporting on AI based primarily on industry press releases or spokespeople. Include sources from outside industry, such as academic researchers, AI ethicists, and others who can offer independent, critical evaluations. When addressing the limitations of AI tools, avoid characterizing non-industry experts as “skeptics.” (This project will develop a working list of individuals and organizations, from outside industry, whom journalists can contact as expert sources on AI.)
- Beware of anthropomorphizing AI systems. Seeking truth and acting transparently and accountably are human activities. By contrast, AI systems are “not designed to seek the truth or engineered to ensure their representations of the world are factual and accurate,” as Bronwyn Jones, Ewa Luger, and Rhia Jones explained in a 2023 risk-based review published by the University of Edinburgh. Likewise, as journalist Hamilton Nolan has argued, “AI lacks the accountability of a human journalist.”
- Avoid characterizing algorithms as inscrutable “black boxes.” In 2018, Joshua Kroll, a computer scientist at UC Berkeley, described algorithms as “fundamentally understandable pieces of technology,” arguing that use of terms such as black box committed a “fallacy of inscrutability.” Journalism committed to algorithmic accountability should hold developers of AI tools accountable for their performance.
- Ask: Does my reporting spread unrealistic expectations (good or bad) about AI? Beware of reporting that adapts either a utopian or dystopian understanding of what AI can do. Focus instead on how AI systems work (or fail to work). What are the challenges posed by this particular use of AI? What guardrails are necessary to make its use safe and equitable?
- Seek opportunities to include the role of AI in stories not otherwise focused on science or technology. For instance, ProPublica’s Machine Bias series illustrates how crime news, a staple for many newsrooms, presents opportunities to address the impacts of AI, including how use of generative AI can reproduce and reinforce existing social inequalities.
This list of practice recommendations will grow and evolve into a robust section of the final resource I’m building — partly through my own work, but also, I hope, based on insights and suggestions from each of you, based on your experience and work.
Please take a moment to complete this brief survey if you would like to suggest what I can build into this resource based on your own work experience.
With declining advertising revenues, staff reductions, and reports that audiences are “disconnected” from news, or only engaging in casual, intermittent “news snacking,” some news organizations are turning, uncritically, to AI in hopes of producing “content” more quickly and less expensively, and boosting its distribution. These trends raise fundamental questions about the role of AI in journalism—which this project, as it develops, will seek to address and provide guidance on how to tackle.
Cite this article
Roth, Andy Lee (2024, July 31). Big tech algorithms: The new gatekeepers. Reynolds Journalism Institute. Retrieved from: https://rjionline.org/news/big-tech-algorithms-the-new-gatekeepers/
Comments