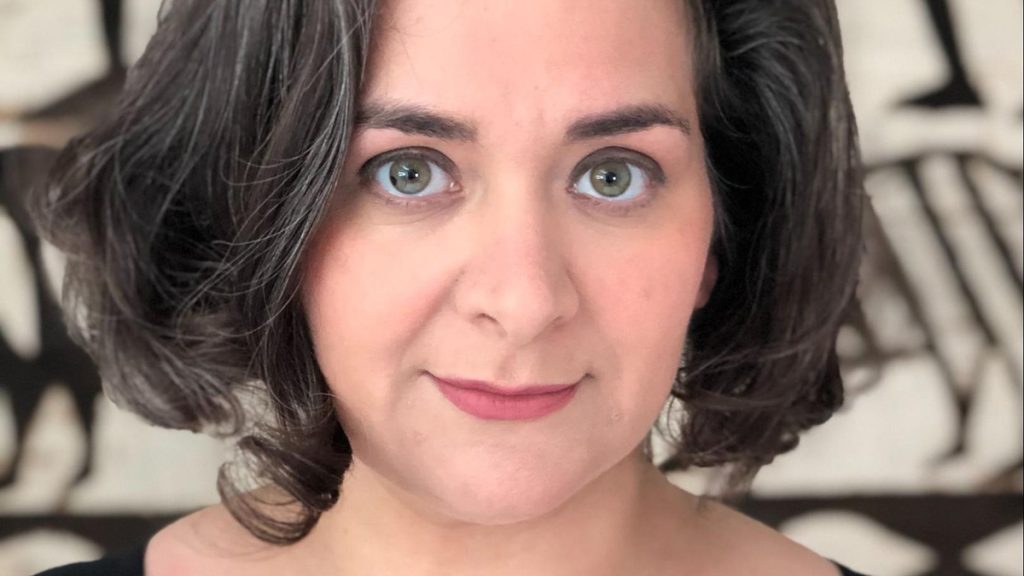
Dr. Keren Landman is a senior reporter for health and science for Vox with a background in public health and internal medicine. She discusses for Innovation in Focus her thoughts on how reporters can give the proper context to data in news coverage.
Contextualizing public health data at Vox
Dr. Keren Landman discusses science literacy, dataset red flags and general tips for science communication
Miesner: Can you tell me about your background and what you do?
Dr. Landman: I am a senior reporter for health and science at vox.com. Between undergrad and med school, I worked in the nonprofit world. I’ve freelanced as a writer. And then I did a lot of work with hospice and I ended up back in the medical world after that and went to med school. I did the EIS program which is the disease detective program at CDC. Basically, an applied epidemiology fellowship and then I worked in public health a little bit at the New York City Health Department, before pivoting to journalism, and freelance for about five years while also working clinically as a pediatrician, before starting to freelance journalism full time.
Miesner: How do you decide what kind of context to provide in your articles?
Dr. Landman: The goal is to allow anybody a way into understanding complicated issues and that means, often starting from the ground up when you’re explaining really complicated health or science topics, and not assuming that anybody knows anything.
That requires sometimes giving people the building blocks of a complicated issue. Without having a scientific background, giving them too much is a tough balance to give somebody enough information to understand a complicated topic without overwhelming them with intricacies that don’t really matter or jargon that is confusing. A lot of what I do and what we at Vox focus on is making sure we’re giving people enough information in a digestible form to allow them to understand the complex.
The work is actually figuring out not only what the science is on something and what is actually happening, but what parts of that background people need to know, to understand what’s happening.
Miesner: How important is science literacy for journalists?
Dr. Landman: I think it’s super important for people to know how science is made, even if you don’t actually focus on science as a beat. Just understanding where science comes from, and that science is designed to make a lot of room for uncertainty.
I think people misunderstand what scientists mean when they say we are almost certain that X causes Y. If you don’t understand that all science is hypothesis driven, and experimentally driven, and sometimes you cannot do certain kinds of experiments, you just have to figure things out observationally.
I think it’s easy to hear a note of uncertainty around certain findings as meaning we don’t actually know or we have no idea and we’re just guessing. In science, citing the biggest scientific discoveries still has a level of uncertainty. Even many of those have some margin of error and people need to understand that that’s because of the way science is done. Not because science cannot actually come up with meaningful answers but because a finding cannot become fact until it is replicated many times.
Miesner: Do you have recommendations on how journalists could be more science literate?
Dr. Landman: I guess you could call it lucky that I spent many years learning how to do science and how to read science before even thinking about writing about science for the press. I didn’t really have to think about how to scale up my knowledge in a short time about something.
There are people coming to do science journalism now who don’t have that privilege. Cramming that kind of understanding into a small into a short period of time is a lot harder. But, there are resources. There are some fellowships geared at doing that. I know the Association of Health Care Journalists has a couple of fellowships aimed at helping journalists read and understand science and learn how science is made.
There are a couple of very good science journalists themselves who write about this and who teach science journalists how to understand intricacies in science, but are learning to do science journalism, in particular, their various programs, whether ranging between a few months.
Miesner: Do you believe that demographic data is necessary for all data in journalism?
Dr. Landman: I think if nothing else, it helps us ensure that first of all, we’re not missing a disparity, and that we’re not missing a reason explaining a disparity.
Not exclusively in the U.S., but especially in the U.S., we have so much systematic bias. Our lack of reckoning with our history of owning people in a race based system has meant our systems have not been cleansed of that racial bias. Looking out for systematic bias allows us to find places where it is hiding. Of course, systematic bias is not just racial bias that we want to be able to unearth in datasets, it’s all kinds of other biases like sexism as well. There are all kinds of ways in which bias makes its way into our systems.
I think it’s super important for people to know how science is made, even if you don’t actually focus on science as a beat. Just understanding where science comes from, and that science is designed to make a lot of room for uncertainty.
If you’re looking for an outcome that you think is based on taking a certain medicine or not, you want to know if everybody has the same access to that medicine. And oftentimes in this country, young people don’t have the same access whether it’s because of income or because of race or other factors.
I think it’s really important to have lots of a variety of different demographic information and often the more the better. One of the more recent kinds of sets of demographic information that we started including is sexual orientation and gender identity.
Miesner: What should journalists do if you have a dataset that is not including demographic data but they feel it’s necessary context for the story?
Dr. Landman: Data is never perfect. Data is never really perfectly clean. And you’re just always going to be missing information. I think the key is to help readers understand what makes a dataset imperfect, what you wish were there, what questions are still remaining because of your data imperfections. Transparency about the flaws in any dataset, I think, is very helpful.
Miesner: How can journalists recognize red flags in a dataset?
Dr. Landman: There’s so many different kinds of datasets, it’s hard to even think about all the kinds of biases that can be built in but I think one of the more common things that we see is that people gather data around an outcome without gathering any control data.
If you learn about what’s happened to folks based on something that’s at the end of a long cascade of events, without looking at any of the people who have not the same cascade of events, whatever those are, then it’s a flawed dataset.
For example, if you want to evaluate stomach aches but only sample people who ate clam chowder for dinner for a week, you might come to the conclusion that everybody who eats clam chowder gets a stomach ache. You haven’t talked to people who ate tomato soup every night or to people who have been eating clam chowder every night for a year.
It’s a really stupid example, but I think people who have not done a lot of science might not intuitively realize that you need to have a control group anytime you’re looking at what caused an outcome. And there’s a reason that we really do need to do that. It’s definitely a red flag if there are no controls.
Generally, the gold standard of a trial that proves something is what’s called a randomized controlled clinical trial or a randomized control trial. If a study is non randomized, if it selects people based on a characteristic often the outcome but not always, and if it’s not controlled, those are signs that a study may not really be proof of anything but that it is really more of a question raising kind of study than an answering study.
Miesner: In your work as a medical reporter, have you found that public health related data needs more contextualization than other types of data that your colleagues may be reporting on?
Dr. Landman: I haven’t spent a ton of time looking at the kinds of data you’re talking about. I do occasionally run across those datasets, but I don’t know nearly as much about them as I do about public health datasets. I am guessing that there’s a lot of messiness in those datasets.
I would generally assume that no data set anywhere is perfect. There may be different mandates for transparency in that world than there are in the medical world, due to the ethics regulations around research. Data that’s often used in public health and in medical sciences, is subject to a level of transparency that I think is really beneficial. You have to explain how you got your data.
It’s very standard to explain how many records were missing from various stages in your data collection, and to show what your groups that you compare were the same or different and to really account for limitations in your data collection.
We need to help people understand how data might be flawed and what direction that might actually push in relation to the actual truth.
Miesner: What types of questions do you believe need to be asked more by journalists when contextualizing data? What steps do you think need to be taken by journalists in order to make data more digestible for readers?
Dr. Landman: I think there are different roles that different types of journalists can play here. Something that I love to see is a good data visualization. The people who make good data visualizations are like, I feel like they are doing the lord’s work, because most of us really cannot internalize and digest a stack of numbers.
There are ways that journalists could do text writing or even radio or audio or video can present numbers, but I think it’s so much easier for most of us to understand numbers visually so the people who are able to visualize data in a way that’s digestible for people. I think that’s a tool that we should embrace and use as much as we can. It’s important to give people not too many numbers.
To help them visualize, the thing you’re talking about is like a layer cake where one layer is composed of these people and the other is different people and if you slice the cake a certain way, you get different types of people. If we can take advantage of familiar ways of understanding to help explain really complicated things I think it really can really help us a lot.
Editor’s Note: This article has been edited for length and clarity by Mikaela Rodenbaugh and Kat Duncan.
Comments