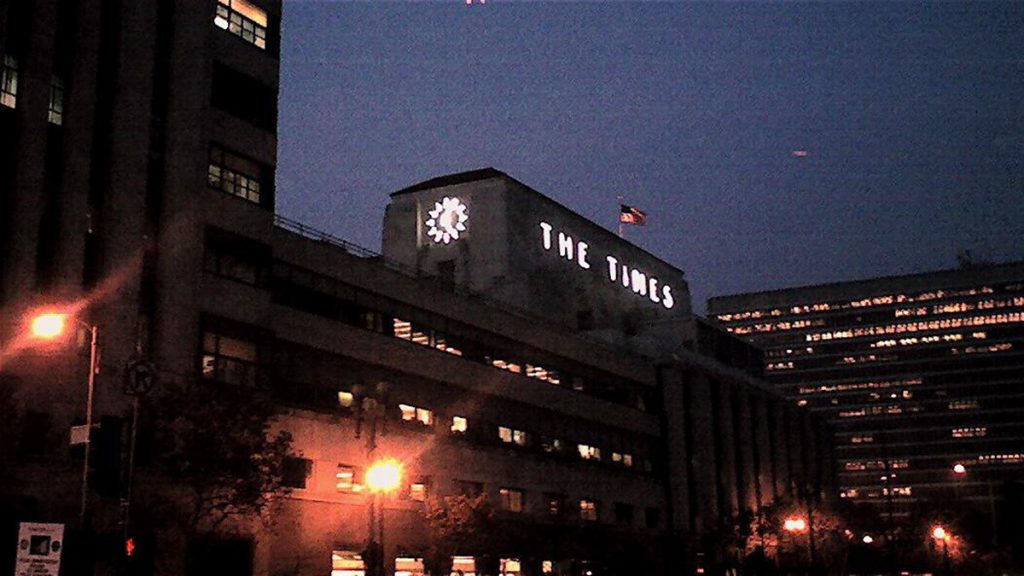
System error
Learning from a newspaper’s plan to deploy an AI-powered “bias meter”
In December 2024, the owner of the Los Angeles Times announced plans to incorporate an AI-powered “bias meter” into the newspaper’s reporting.
If the newspaper’s owner, medical doctor and biotech billionaire Patrick Soon-Shiong, was serious—and not simply firing a shot across the bow of his newspaper’s unionized staff—his plan reflects flawed understandings of journalistic ethics and the potential for AI-powered technologies to reproduce, and potentially amplify, social inequalities and risks.
Soon-Shiong discussed the tool during a friendly interview with CNN’s Scott Jennings on the Flyover Country podcast. Presenting himself as a protector of journalistic balance, intent on making the Los Angeles Times a “trusted source,” Soon-Shiong revealed the development, “behind of the scenes,” of a “bias meter” that would use “augmented intelligence” to inform readers about the “level of bias” in any news story. He expressed hope that the meter would launch in January. Following the interview with Jennings, Soon-Shiong backtracked, clarifying that the meter would rate editorials and opinion columns but not news stories.
The day after Soon-Shiong’s podcast appearance, the Los Angeles Times Guild, which represents more than 300 editorial employees at the newspaper, expressed outrage that “the newspaper’s owner has publicly suggested his staff harbors bias without offering evidence or examples.”
Major news organizations—including the New York Times, CNN, Forbes, and NPR—covered Soon-Shiong’s announcement. Many linked it to prior resignations of several of the newspaper’s editorial board members and senior staff after Soon-Shiong blocked the board’s decision to endorse Kamala Harris in the 2024 presidential election.
When this article was written in mid-January, there were no further reports on plans to activate Soon-Shiong’s “bias meter” at the Los Angeles Times. However, even if nothing comes of this specific proposal, examining three flawed assumptions embedded in Soon-Shiong’s scheme can help clarify future debates about AI’s role in vetting bias in news reporting.
News is never a simple reflection of a fixed reality
News slant is one of many problems technology boosters have proposed to address using algorithmic-powered tools. Proponents argue that tools like Soon-Shiong’s “bias meter” could make judgments of news bias objective and fair.
“Calling this tool a ‘bias meter’ is a misnomer,” Sisi Wei, the Chief Impact Officer at The Markup and CalMatters, explained. “It’s more of an ‘ideology classifier,’ which gets around tougher questions that a ‘bias meter’ would really create, such as what counts as biased information and to whom?”
News bias is fundamentally resistant to assessment by automated systems because interpretation—often glossed as “news judgment”—is intrinsic to reporting. To paraphrase sociologist Harold Garfinkel, seeking to rid journalism of interpretation is akin to suggesting that, if only we could get rid of a building’s walls, we could see more clearly what is keeping the roof up.
But could something like a bias meter help readers understand how reporters’ news judgment shapes the production of their stories? Again, there are grounds for skepticism.
Trustworthy outputs require concrete explanations, not simplistic metrics
Noting that a “bias meter” of the sort floated by Soon-Shiong would shift power from journalists to whoever is developing or managing the tool, Jack Bandy, a computer scientist at Transylvania University, expressed doubt about rating articles on an “oversimplified five-point scale of left/right political bias,” without providing “concrete explanations” of the model’s output.
Bandy—who has written about using natural language processing to measure political bias in tweets, speeches, and articles by counting the frequency of partisan phrases—described how more specific explanations could show links between rhetoric and bias. For example, he suggested a tool might offer an explanation such as, “This article uses the term ‘illegal alien’ four times, which is more common in anti-immigrant rhetoric.” Bandy indicated that a tool that provided explanations of this sort would be more useful.
However, Bandy also raised the possibility that widespread reader reliance on such a tool could lead to a “paradox of automation,” in which the system’s perceived efficiency actually makes the human contributions of its operators more crucial.
Exclusive algorithms thwart transparency, public trust
Proposals such as Soon-Shiong’s ostensibly seek to renew public trust in journalism. But, as Federico Guerrini of Forbes noted, audience distrust of news outlets is “actually fuelled and amplified by technology.” Similarly, NPR’s media correspondent, David Folkenflik, told All Things Considered that an AI-driven bias meter would be “a legal disaster,” inviting lawsuits against the newspaper, a scenario unlikely to convince readers of a newspaper’s credibility.
“In a climate where the lines in the sand between news and opinions can easily be muddled, public trust in journalism is critical,” Emily Bloch, president of the Society of Professional Journalists, acknowledged. However, noting that the Los Angeles Times’s owner provided no evidence of his staff’s bias and that AI “can produce its own factual errors,” Bloch said, “I have sincere doubts that a ‘bias meter’ will be the solution here.”
Soon-Shiong’s proposal exemplifies what Princeton-based computer scientists Arvind Narayan and Sayash Kapoor call AI snake oil, artificial intelligence that “does not and cannot work as advertised.” Any AI-powered “bias meter” will almost certainly reflect the biases of the existing materials on which it has been trained, if not those of its developers. But, as Narayan and Kapoor note in their 2024 book, AI Snake Oil, developers have few incentives for transparency. Invoking trade secrets, they avoid making their models available for public scrutiny. Narayan and Kapoor explain that this is compounded by the conventional practice of determining an AI tool’s validity through use of benchmark datasets, which are often poor proxies for real-world utility.
Ninety-four percent of the public want journalists to disclose their use of AI, according to a 2024 survey by Trusting News and the Online News Association. But simple disclosure is only a small first step toward promoting journalistic transparency and public trust. Public trust will hinge on understanding how, not just whether, newsrooms are using AI.
Unlike reporters and editors, who can explain how they developed a story if asked, including, for example, what sources they used and why one angle was made primary when another was not, AI tools cannot provide accounts for the processes that lead to their outputs.
A tool of the sort floated by Soon-Shiong might become the first step in a leap forward for data-driven journalism, galvanizing public support at a fateful juncture in the history of the profession. But the true potential of any such innovation to serve the public good—rather than exclusive political or economic interests—will demand serious consideration by editors and reporters, in addition to owners and shareholders, of the complex balancing act between new technologies, editorial autonomy, and professional ethics.
Cite this article
Roth, Andy Lee (2025, Feb. 4). System error. Reynolds Journalism Institute. Retrieved from: https://rjionline.org/news/system-error/
Comments