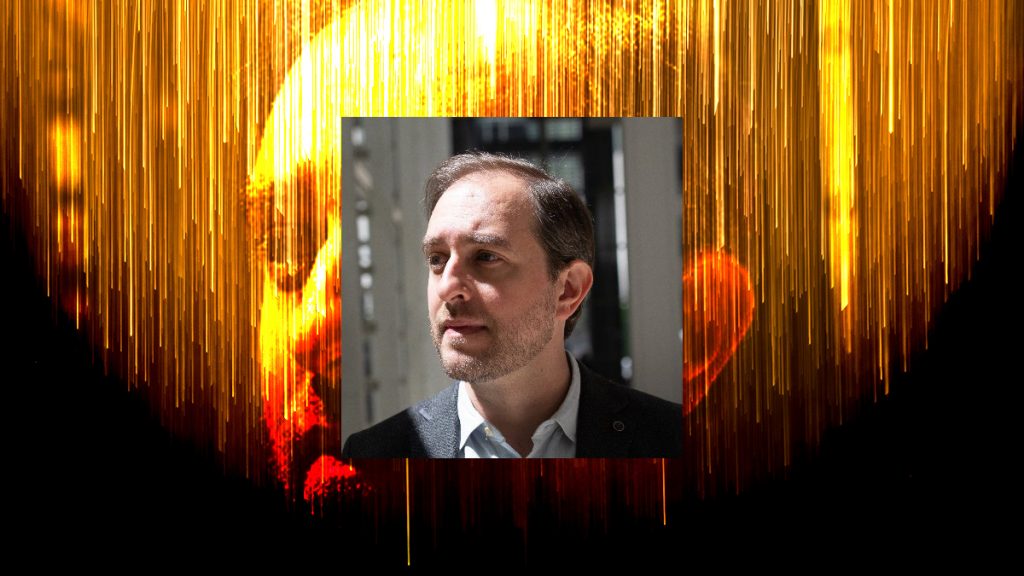
Portrait courtesy Marc Lavallee | Background image: Rene Böhmer | Unsplash
When and how should we use AI in news?
We spoke with Marc Lavallee of The New York Times about newsrooms incorporating AI technology and tools into their workflow
For Innovation in Focus earlier this month, I tested an AI editing software while reporting on a story for the Columbia Missourian to see how it could be incorporated and used as an editor to complement or replace human editing. For this piece, I spoke with Marc Lavalle, the director of Research and Development at The New York Times, about AI’s role in news and how it can be used in various journalistic processes.
Doheny: How do you decide when and what AI technology to use?
Lavallee: When AI solves a tangible need in a way that is visible to a journalist. Then the fact that it’s AI or machine learning, is almost incidental. People don’t use Trint because it’s AI, people use Trint because they get a pretty good copy of a transcript pretty fast. And so the things that take root for journalism are things that I think check that box. When it comes to the effectiveness of a tool, whether or not it claims it’s AI or not, and whether or not it truly is AI or not, falls short of: Does it deliver value to us?
Doheny: What are some examples of how the Times has incorporated AI?
Lavallee: A lot of what’s relevant is the natural language processing area. One of the ways that we’ve invested a lot of time in really trying to build something relatively unique, is in the ability to answer readers’ questions. The way that we’re trying to approach that is predicated on a belief that, in a lot of situations, we’ve probably already written something that answers the question that the reader is asking. The common format is a FAQ page kind of thing.
So what we focused on there is the ability for you to ask a question, using your own words, that can be matched to the way that we wrote the question-answer pair. So if I asked: Is air travel safe? And you said: Is it okay to fly? There, aside from the word is, no other words match up there. What we tried to do is, train a model to be able to understand many ways that a person would ask a question that is fundamentally the same question, and be able to get the answer using their own language. That is story level execution, to be able to put something in the real world and be able to test it out.
Another big area, which is much more visual, is in taking techniques of computer vision, and figuring out how to apply them more to the reporting process. A lot of our specific examples have been in sports. To be able to look at videos or still photography from sporting events, and be able to have a much better understanding of what’s going on. You can think about the height a gymnast jumps on a balance beam, or the velocity that they move through the air.
We did this with the Tokyo Olympics around swimming and track and field events to get much more precise. It gives us a more detailed way of being able to explain things than just, 10 people run very quickly to the finish line, and two of them kind of look like they’re a little bit out ahead. To be able to say, if we can answer this question authoritatively, how would we explain that? Where would we use this? How would we visualize that?
Doheny: How difficult or easy is it to incorporate these technologies into the daily workflow of a newsroom?
Lavallee: It’s hard. When working with novel technologies, there may be more risks to account for and many fewer precedents. There’s a lot more work beyond just the pure technical implementation to make sure that you’re doing something where essentially that risk benefit balance is appropriate and within the standards and guidelines that we aspire to for journalism at large.
And I think that there’s the question of when the New York Times uses a technology, is there some level of endorsement of that technology or exposure of that technology? Which is an open question of even if we at a particular instance use something that checks all the boxes, and it’s within our standards and ethics, is there a normalization of some of these technologies by media organizations that has a greater societal effect? And how do we deal with that? That’s still a big open question.
Doheny: How can a small newsroom with less resources include AI technology?
Lavallee: When it comes to AI, I think one thing to look at is, who and what tools exist out there that are usable by an overlap between our industry and other industries. Trint, the transcription program, was created by people who previously worked at BBC. And so they really understand journalists’ needs. But that is a tool that is useful for a whole lot of people, not just journalists.
Doheny: Do you ever find that AI doesn’t work like you expected?
Lavallee: It’s almost like this two by two grid, like an approval matrix: did it do something useful for us? And, is it actually AI? The usefulness axis is really important to us. The AI axis kind of isn’t. I would say, the place where we try to be very careful is that we’re not here to evangelize a certain kind of technology or a certain kind of solution or approach.
Things don’t work all the time. It isn’t a, this doesn’t work, therefore, it’ll never work. It’s like, it doesn’t work yet. So a lot of the questions for us are more about timing than anything else. I think the biggest gaps that we try to manage are between where our readers already are, and where our perceptions of them are. And really be able to use behavior as an indicator.
Doheny: What have you learned as you’ve worked with AI technology?
Lavallee: There is this exponential increase in the efficacy of a lot of these underlying bits of AI and machine learning. I think we’ve learned that for a tool to be useful to a journalist or to a person, so many things have to come together in a lot of these situations. We can collectively have a decent understanding of what we want these technologies to do for us and even though aspects of them are improving rapidly, they can still take a really long time.
For news organizations trying to figure out how to adopt emerging technologies, I think a big thing we’ve learned is trying to predict that timing pretty far out takes a lot of work. And it’s very easy to be wrong there.
Editor’s note: This interview has been edited for length and clarity.
Comments